Quantifying Uncertainty: David Kaplan Explains the Usefulness of Bayesian Statistics
June 23, 2014
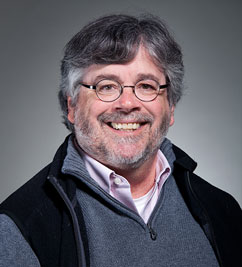
David Kaplan
To most academics, and even many statisticians, the world of Bayesian statistics remains a dark and dangerous realm, rarely visited and greatly feared.
To David Kaplan, Bayesian statistics are enlightening, capable of bringing clarity to difficult problems. But as much as Kaplan, a professor of quantitative methods and chair of the Department of Educational Psychology at UW–Madison, advocates for the increased use of Bayesian methods in quantitative research, he warns that researchers should make their initial forays with caution.
“Thanks to greater computing power, Bayesian calculations can now be completed even on a tablet, which can give researchers a powerful tool for gaining new insights from their data analyses,” Kaplan said. “However, there are major differences in paradigms and methodologies that need to be understood before just plugging data into that fancy new software on your iPad and putting the results in a research paper.”
A new book by Kaplan, Bayesian Statistics for the Social Sciences, scheduled for publication in mid-August, introduces the Bayesian model to those who are more familiar with the classical, “frequentist” approach, in which a hypothesis is tested without quantifying one’s degree of uncertainty about the hypothesis. Bayesian statistical methods begin by quantifying one’s uncertainty on an issue, which is then updated in light of new data. Ideally, over time, one’s uncertainty about a problem diminishes.
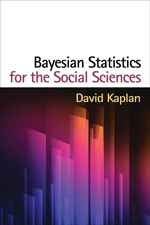
“Right now, Bayesian statistics is the shiny new object. Once technology made it possible to run Bayesian analysis, there was an explosion of interest in using it,” Kaplan said. “But before jumping in head-first, it’s important for researchers to understand how it differs from the classical perspective and what advantages and disadvantages that different approach creates.”
Kaplan, an inveterate Chicago Cubs fan, likes using professional baseball to clarify the paradigmatic differences between the two statistical approaches.
“The classical (frequentist) view sees the probability of the Cubs winning the World Series entirely based on their past frequency of winning the World Series – which, since 1908 is zero,” Kaplan said. “The Bayesian view definitely incorporates past performance but, in addition, uses what is known about the Cubs—such as their current pitching roster or the on-base percentage of their line-up—to provide a different probability. In other words, I know the Cubs haven’t won the World Series since 1908, but that is only one piece of information that should be used in betting on the Cubs.”
Many of the examples Kaplan uses in his book come not from baseball but from the world of education. Kaplan is an expert on the Programme for International Student Assessment (PISA), as he formerly served as a member of the PISA Technical Advisory Group and currently chairs the PISA Context Questionnaire Expert Group. Kaplan draws from his familiarity with PISA, which tests millions of 15-year-olds from dozens of countries on math, science, and reading, to elucidate his Bayesian lessons.
Using the 2009 PISA to study predictors of reading literacy among 15-year-olds in the United States, Kaplan said a classical approach would not consider any other data besides that single assessment. With a Bayesian approach, however, Kaplan said his statistical model could include results from the 2000 PISA, which collected similar data on reading literacy in the United States. That additional information could give him a better idea of the actual literacy performance of American 15-year-olds at the time the test was given.
“It’s a method of learning from prior information,” Kaplan said. “I like to refer to it as ‘evolutionary knowledge development.’”
A frequent criticism of Bayesian theory is that allowing statisticians to incorporate their subjective beliefs might unintentionally skew their results toward their desired outcome. But Kaplan acknowledges the merit of that argument, but contends that it’s not a big issue, since a true Bayesian approach identifies all prior beliefs clearly, allowing critics to test for and identify bias on a case-by-case basis.
“It’s impossible to escape the subjectivity, but you can at least warrant your claims with empirical data,” Kaplan said.
With big datasets like those from the PISA, Kaplan conceded there wouldn’t be much difference between the results of a Bayesian or classical approach. Where a Bayesian model really comes in handy is with small datasets, he explained.
“In neuropsychology, researchers can’t run 5,000 people through a magnetic resonance imaging machine. Maybe 20 is the max they can actually support on a grant. But to do a classical analysis with a sample size of 20 would be next to impossible,” Kaplan said. “In small batches, integrating your prior beliefs can make quite a difference. You can factor in a whole lot of information collected in other studies to help shape your analysis.”
Bayesian Statistics for the Social Sciences is a hybrid book, offering both an overview of the current state of the field of statistics and specific examples of Bayesian methodology put to practical use. In writing such a book, Kaplan said he aimed to cast a wider net for potential readers. His peer reviewers agreed that anyone with an interest in the subject will find the book interesting, but Kaplan admits it is geared toward graduate students in the social sciences with at least a background in statistics, in fields such as sociology, psychology, population health, or educational psychology.
His message has already found an eager audience. Kaplan has done presentations and workshops all over the world, with recent stops at the National Taichung University of Education in Taiwan, the Fourth Congress on Measurement and Evaluation in Education and Psychology in Ankara, Turkey, and an upcoming set of lectures at the European Union’s Joint Research Center in Ispra, Italy.
“Computing power has made Bayes the hot new thing, opening up a brand new world for researchers,” Kaplan said. “I see it as my job to make sure they enter it with their eyes open.”