Value-Added Measurement: What It Is and Is Not
December 15, 2012
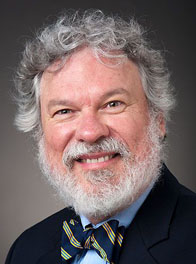
Rob Meyer
Whether we’re measuring teacher skills or school performance, value-added evaluation continues to shape our definition of successful education.
According to researchers at the Value-Added Research Center (VARC) at UW–Madison, a value-added model is simply a statistical formula that estimates the contribution of schools, classrooms, teachers, and other educational factors to student achievement. What makes value-added unique is that it also measures, and controls for, non-school sources of student achievement growth, including, for example, family education, social capital, and household income. Value-added models take into account that different schools serve very different populations of students.
Controlling for non-school influences allows educators and researchers, like those at VARC, to make apples-to-apples school comparisons rather than apples-to-oranges comparisons.
Value-added measurement provides one way to help determine the effectiveness of teachers and schools at the K–12 level and in postsecondary institutions.
For example, Education Week reports that more than a dozen states plan to use value-added measurement to analyze how graduates of teacher education programs fare in their real-world classrooms. The data may help determine which teacher education pathways produce teachers who are at least as good as—or even better than—other novice teachers, spurring other providers to emulate these practices.
Yet value-added evaluation stirs controversy. In large part that’s because it has been misunderstood or misused.
In 2010 the Los Angeles Times published teachers’ value-added scores and their names, causing a widespread outcry. The available data reflected only teacher performance as it relates to students’ performance on standardized test scores, which is only one factor in rating a teacher’s performance and, taken alone, produces a distorted picture. The Times warned that huge margins of error surround the ratings: more than 30 percentile points in math and more than 50 percentile points in English language arts.
Educator Linda Darling-Hammond reacted strongly against what she called “the tabloid treatment” that singled out those teachers. The Stanford University education professor says using “value-added methods” can be valuable for large-scale studies, but calls the methods seriously flawed for evaluating individual teachers.
In February 2012 New York City’s Department of Education released value-added scores to news outlets, several of which plan to make the data publicly available. The United Federation of Teachers has tried to prevent the release.
Philanthropist Bill Gates is among those arguing that making these reports public amounted to a "public shaming" of teachers that could threaten reform in how teachers are evaluated.
A recent analysis by economists at Harvard and Columbia universities found that having a high-quality teacher for even 1 year can have a measurable long-term impact on students’ career outcomes. The study tracked 1 million children from a large urban school district from fourth grade to adulthood. The researchers gauged the effectiveness of their teachers in grades 4 through 8 through value-added analysis, calculating their impact on student standardized test scores over time, making adjustments for differences in student characteristics. The researchers found that students who were assigned teachers with higher value-added ratings ended up being “more successful in many dimensions,” including college graduation rates, earnings, and savings.
Those who research, develop, and help administer value-added evaluations say that the most complete picture of student and school performance results from considering both student achievement scores and value-added measures. This combined approach shows what students know at a point in time (achievement) and how the school affects student academic growth (value-added).
How does value-added evaluation work?
At the teacher level: Analogy of the two gardeners
The “two gardeners” analogy is sometimes used to introduce the concept of value-added measurement.
Imagine we wish to compare the performance of two gardeners who grow oak trees, Gardener A and Gardener B. Each works in different conditions of soil quality, sunlight, rainfall, and other environmental factors.
First, we measure the oak tree heights 1 year after the gardeners began tending them. With a height of 61 inches for oak tree A and 72 inches for oak tree B, Gardener B seems to be the better gardener.
Second, we compare the height of the trees 1 year ago to the height today. We find that Gardener A’s tree grew 14 inches while Gardener B’s tree grew 20 inches. Because oak B had more growth this year, Gardener B seems better. In terms of measuring student achievement, this is analogous to using a “simple growth model,” also called student gain.
Say that a botanist had predicted tree growth based on average rainfall, soil quality, and temperatures over the year. The botanist predicted that a tree growing in oak A’s conditions would be 59 inches, but it’s now 61. The predicted height for trees in oak B’s conditions was 74 inches, but now it’s only 72. Using this method, Gardener A now seems to be the better gardener.
By accounting for last year’s tree height and for environmental conditions during this year, we have found the “value” each gardener “added” to tree growth. This is analogous to a value-added measure in education.
Let’s consider two other gardeners, C and D. They will care for trees C and D for the next year. How can we be fair to these gardeners using a value-added model?
We might ask whether tree height might have an effect on tree growth. Tall trees would represent high-achieving students; short trees would represent low-achieving students. Some short trees grow more quickly than tall trees. Would gardeners with short trees enjoy an advantage?
In the same way that we measured the effect of rainfall, soil richness, and temperature, we can determine the effect of prior tree height (student achievement) on growth.
We then collect data on all oak trees in this region and measure whether short or tall trees grew faster. In this case, we determine that short trees tended to grow more in a given year.
In the earlier analogy, we assumed that all trees grew 20 inches during a year of care and then refined our predictions with each tree’s environmental conditions. By including prior height in the model, we can improve our predictions by taking this data into account.
When measuring student achievement it’s been found that students who are already high achievers tend to gain fewer points during a single year of growth. By controlling for this trend when we make predictions, value-added estimates can fairly compare the growth of students from across the achievement spectrum.
At the district level
Now let’s look at three schools serving different student populations. For example, School A serves mostly students with very high test scores. On the other extreme, School C serves mostly students with very low test scores.
Value-added measurement analyzes the trend of scores for all students in a district or state. That enables one to determine the appropriate adjustments to counteract the effects of School A, School B, and School C, in predictions.
After we make these adjusted predictions, we can fairly evaluate the growth of students in schools serving students with any achievement level distribution—low, high, or average. We can compare high-achieving students in School A to typical growth for high-achieving students from across the district or state. Likewise, we can compare low-achieving students in School C to typical growth for similar low-achieving students from across the district or state.
How does VARC choose what to control for?
VARC requires that a characteristic must meet several requirements before it will be considered for inclusion in the value-added model:
Check 1: Is this factor outside the teacher’s influence?
Check 2: Do we have reliable data?
Check 3: If we lack reliable data, can we approximate it with other data?
If there is a detectable difference in growth rates for different groups of students, we attribute this to a challenge to be addressed at the district or state level, not something an individual teacher or school should be expected to overcome individually. If a particular school, grade-level team, or teacher is making above-average results with any group of students, this will be reflected in an above-average value-added estimate.
Researchers use all the data available to get the most complete picture of the real situations of students and to make as accurate a prediction as possible. The more complete job value-added can do to control for external factors, the more accurately researchers can evaluate the effect of districts, schools, grades, classrooms, programs, and interventions.
For an introductory video hosted by VARC Director Rob Meyer see http://varc.wceruw.org/tutorials/meyer/Robs_Welcome.html
For more information about the Value-Added Research Center, its methodologies, staff, and tutorial products, see http://varc.wceruw.org/